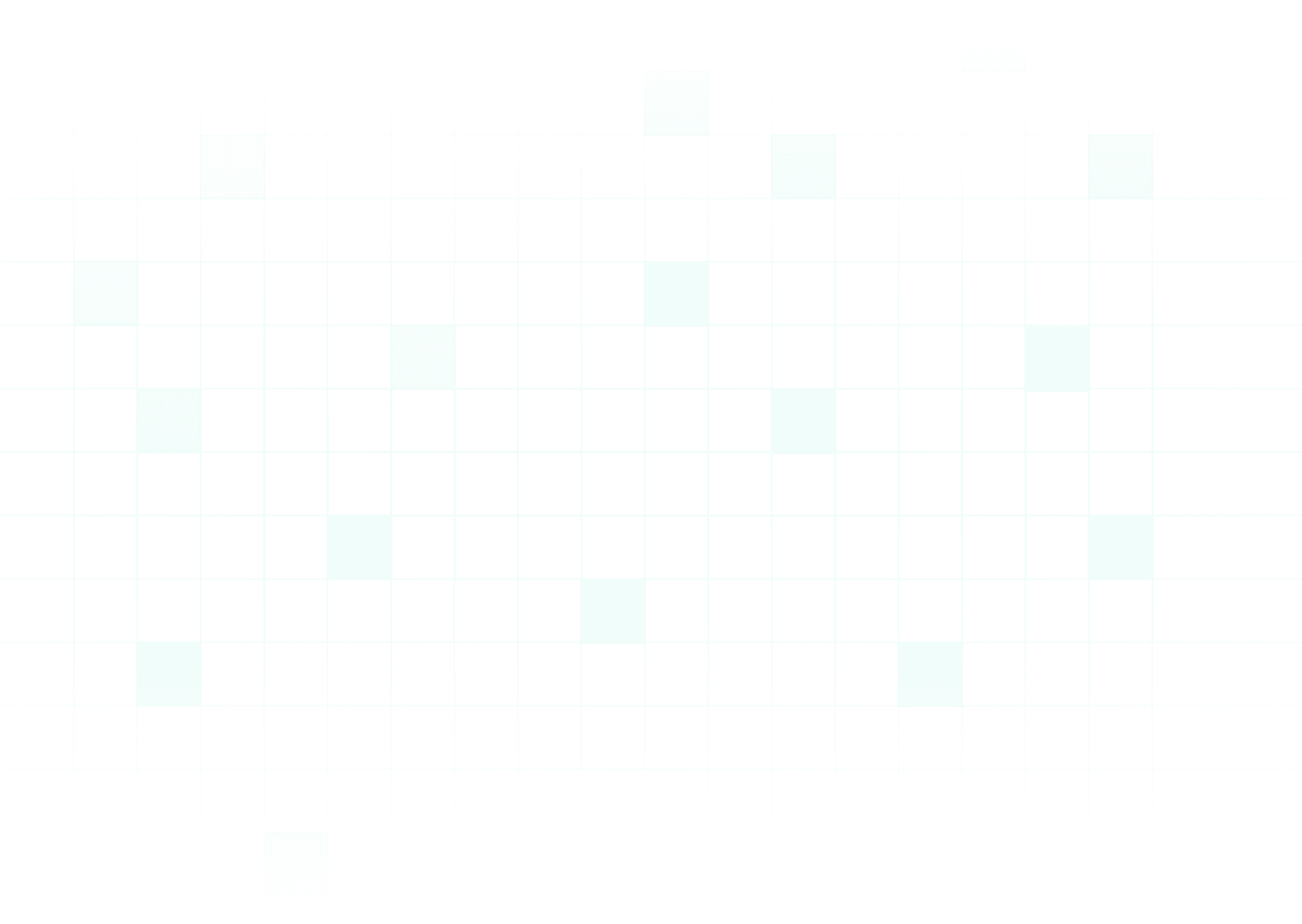
Proactive Strategies for Preventing and Mitigating AI Hallucinations
Proactive Strategies for Preventing and Mitigating AI Hallucinations
Proactive Strategies for Preventing and Mitigating AI Hallucinations
Nov 21, 2023


As artificial intelligence advances, we must address new challenges, such as AI hallucinations. Here, we discuss some essential methods, such as using diverse training data, improving model interpretability, and implementing robust testing procedures that reduce the likelihood of this phenomenon.
Diversifying Training Data
One of the primary reasons AI hallucinates is limited and biased training data, causing incorrect patterns and false interpretations.
To mitigate this, we should use diverse and comprehensive training data. The accuracy of AI interpretations improves with more representative data.
Improving Model Interpretability
Another strategy is to improve the interpretability of AI models. Interpretability refers to our ability to understand how the AI makes its decisions. By constructing more interpretable models, we can better understand when and why the AI is likely to hallucinate. This can help us identify potential issues before they become problematic and make necessary adjustments to the AI's training.
Robust Testing Procedures
Implementing robust testing procedures is another critical strategy. This involves testing the AI in various scenarios and conditions to see how it behaves. If the AI starts to hallucinate, the testing procedures can help identify the problem early on. We can then take steps to correct the issue, whether that means adjusting the AI's training, tweaking the model, or even redesigning the system entirely.
Continuous Monitoring and Updating
Continuous monitoring and updating of AI systems are paramount to ensuring expected performance. If an AI system starts to hallucinate, continuous monitoring can help catch this early on so that necessary adjustments can be made. Regular updates also allow the system to learn from new data and adapt to changing conditions, reducing the likelihood of hallucinations.
Building Ethical Guidelines
Establishing ethical guidelines for AI use is also an important step. These guidelines help ensure that AI systems are used responsibly, with due consideration for potential risks like hallucinations. They can also provide a roadmap for addressing any issues, ensuring they are dealt with promptly and effectively.
Keep on Learning with Aivia
To know more about these strategies, Aivia encourages you to visit their Professional Development Center. There, you can watch the course module "Human-in-the-Loop," which provides insights into the role of human involvement in preventing and mitigating AI hallucinations.
As artificial intelligence advances, we must address new challenges, such as AI hallucinations. Here, we discuss some essential methods, such as using diverse training data, improving model interpretability, and implementing robust testing procedures that reduce the likelihood of this phenomenon.
Diversifying Training Data
One of the primary reasons AI hallucinates is limited and biased training data, causing incorrect patterns and false interpretations.
To mitigate this, we should use diverse and comprehensive training data. The accuracy of AI interpretations improves with more representative data.
Improving Model Interpretability
Another strategy is to improve the interpretability of AI models. Interpretability refers to our ability to understand how the AI makes its decisions. By constructing more interpretable models, we can better understand when and why the AI is likely to hallucinate. This can help us identify potential issues before they become problematic and make necessary adjustments to the AI's training.
Robust Testing Procedures
Implementing robust testing procedures is another critical strategy. This involves testing the AI in various scenarios and conditions to see how it behaves. If the AI starts to hallucinate, the testing procedures can help identify the problem early on. We can then take steps to correct the issue, whether that means adjusting the AI's training, tweaking the model, or even redesigning the system entirely.
Continuous Monitoring and Updating
Continuous monitoring and updating of AI systems are paramount to ensuring expected performance. If an AI system starts to hallucinate, continuous monitoring can help catch this early on so that necessary adjustments can be made. Regular updates also allow the system to learn from new data and adapt to changing conditions, reducing the likelihood of hallucinations.
Building Ethical Guidelines
Establishing ethical guidelines for AI use is also an important step. These guidelines help ensure that AI systems are used responsibly, with due consideration for potential risks like hallucinations. They can also provide a roadmap for addressing any issues, ensuring they are dealt with promptly and effectively.
Keep on Learning with Aivia
To know more about these strategies, Aivia encourages you to visit their Professional Development Center. There, you can watch the course module "Human-in-the-Loop," which provides insights into the role of human involvement in preventing and mitigating AI hallucinations.
As artificial intelligence advances, we must address new challenges, such as AI hallucinations. Here, we discuss some essential methods, such as using diverse training data, improving model interpretability, and implementing robust testing procedures that reduce the likelihood of this phenomenon.
Diversifying Training Data
One of the primary reasons AI hallucinates is limited and biased training data, causing incorrect patterns and false interpretations.
To mitigate this, we should use diverse and comprehensive training data. The accuracy of AI interpretations improves with more representative data.
Improving Model Interpretability
Another strategy is to improve the interpretability of AI models. Interpretability refers to our ability to understand how the AI makes its decisions. By constructing more interpretable models, we can better understand when and why the AI is likely to hallucinate. This can help us identify potential issues before they become problematic and make necessary adjustments to the AI's training.
Robust Testing Procedures
Implementing robust testing procedures is another critical strategy. This involves testing the AI in various scenarios and conditions to see how it behaves. If the AI starts to hallucinate, the testing procedures can help identify the problem early on. We can then take steps to correct the issue, whether that means adjusting the AI's training, tweaking the model, or even redesigning the system entirely.
Continuous Monitoring and Updating
Continuous monitoring and updating of AI systems are paramount to ensuring expected performance. If an AI system starts to hallucinate, continuous monitoring can help catch this early on so that necessary adjustments can be made. Regular updates also allow the system to learn from new data and adapt to changing conditions, reducing the likelihood of hallucinations.
Building Ethical Guidelines
Establishing ethical guidelines for AI use is also an important step. These guidelines help ensure that AI systems are used responsibly, with due consideration for potential risks like hallucinations. They can also provide a roadmap for addressing any issues, ensuring they are dealt with promptly and effectively.
Keep on Learning with Aivia
To know more about these strategies, Aivia encourages you to visit their Professional Development Center. There, you can watch the course module "Human-in-the-Loop," which provides insights into the role of human involvement in preventing and mitigating AI hallucinations.