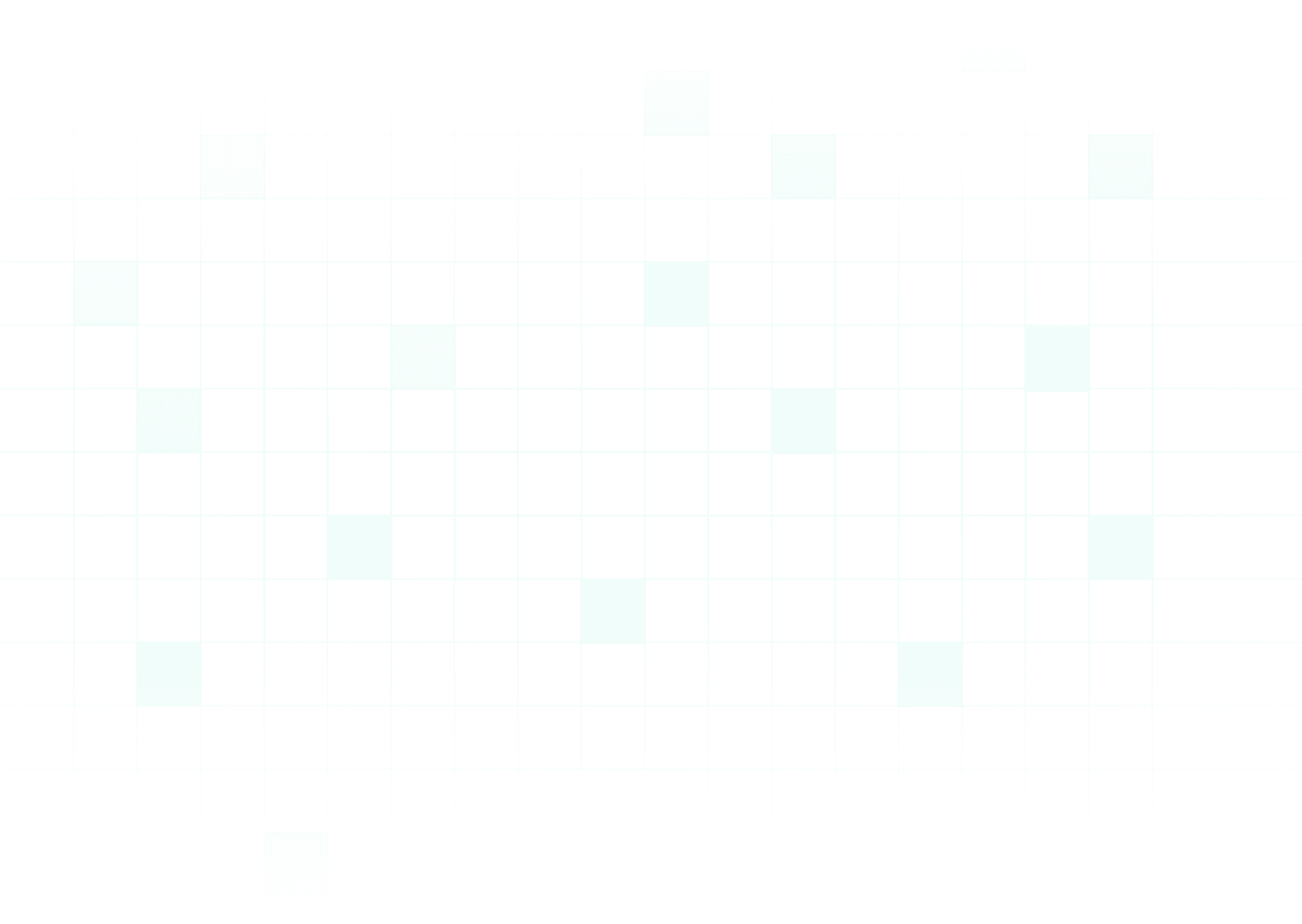
AI Hallucinations: Understanding the Factors Behind AI System Errors
AI Hallucinations: Understanding the Factors Behind AI System Errors
AI Hallucinations: Understanding the Factors Behind AI System Errors
Nov 21, 2023


AI hallucination is a complex phenomenon influenced by multiple factors. While it’s often simplified as a “misinterpretation of training data,” the reality is more nuanced. In this text, we’ll explore the root causes of AI hallucinations, such as overfitting, biased training data, and misinterpretation.
Insufficient, Outdated, or Low-Quality Training Data
The quality of an AI model’s training data significantly influences its performance. If the model is trained with insufficient, outdated, or low-quality data, it may struggle to generate accurate, relevant responses. It might hallucinate by producing outputs based on patterns it thinks it has identified in its training data, but these patterns may not accurately represent the task at hand or the real-world context.
Overfitting
Overfitting is a common issue in machine learning where a model performs well on its training data but poorly on new, unseen data. Overfit models are typically complex, with many parameters fine-tuned to capture noise or random fluctuations in the training data. This results in memorizing the training data and failing to generalize to new data.
Biases in Training Data
Bias in training data is another significant factor that can lead to AI hallucinations. If the data used to train an AI model is biased, the model will likely reproduce and amplify these biases in its responses. For instance, if a language model is trained on text data that over-represents a particular demographic group, it might generate biased outputs that favor that group.
Model Misinterpretation
Finally, model misinterpretation can also cause AI hallucinations. This can occur when an AI system incorrectly interprets the patterns it identifies in its training data. For example, a model might identify a correlation in its training data and interpret this as a causative relationship, leading to inappropriate or inaccurate outputs.
It is essential to comprehend the reasons behind AI hallucinations as they are pivotal in developing more dependable and trustworthy AI systems. By dealing with issues like data quality, bias, overfitting, and model interpretation, we can reduce the danger of hallucinations and augment the performance of AI systems.
Aivia's Approach to Understanding and Mitigating AI Hallucinations
To prevent hallucinations in AI-generated content, it is crucial to have a human supervise the process during both training and content creation. To learn more about the human-in-the-loop approach to mitigating AI systems’ hallucinations, ensure to watch our video, which you can find here.
AI hallucination is a complex phenomenon influenced by multiple factors. While it’s often simplified as a “misinterpretation of training data,” the reality is more nuanced. In this text, we’ll explore the root causes of AI hallucinations, such as overfitting, biased training data, and misinterpretation.
Insufficient, Outdated, or Low-Quality Training Data
The quality of an AI model’s training data significantly influences its performance. If the model is trained with insufficient, outdated, or low-quality data, it may struggle to generate accurate, relevant responses. It might hallucinate by producing outputs based on patterns it thinks it has identified in its training data, but these patterns may not accurately represent the task at hand or the real-world context.
Overfitting
Overfitting is a common issue in machine learning where a model performs well on its training data but poorly on new, unseen data. Overfit models are typically complex, with many parameters fine-tuned to capture noise or random fluctuations in the training data. This results in memorizing the training data and failing to generalize to new data.
Biases in Training Data
Bias in training data is another significant factor that can lead to AI hallucinations. If the data used to train an AI model is biased, the model will likely reproduce and amplify these biases in its responses. For instance, if a language model is trained on text data that over-represents a particular demographic group, it might generate biased outputs that favor that group.
Model Misinterpretation
Finally, model misinterpretation can also cause AI hallucinations. This can occur when an AI system incorrectly interprets the patterns it identifies in its training data. For example, a model might identify a correlation in its training data and interpret this as a causative relationship, leading to inappropriate or inaccurate outputs.
It is essential to comprehend the reasons behind AI hallucinations as they are pivotal in developing more dependable and trustworthy AI systems. By dealing with issues like data quality, bias, overfitting, and model interpretation, we can reduce the danger of hallucinations and augment the performance of AI systems.
Aivia's Approach to Understanding and Mitigating AI Hallucinations
To prevent hallucinations in AI-generated content, it is crucial to have a human supervise the process during both training and content creation. To learn more about the human-in-the-loop approach to mitigating AI systems’ hallucinations, ensure to watch our video, which you can find here.
AI hallucination is a complex phenomenon influenced by multiple factors. While it’s often simplified as a “misinterpretation of training data,” the reality is more nuanced. In this text, we’ll explore the root causes of AI hallucinations, such as overfitting, biased training data, and misinterpretation.
Insufficient, Outdated, or Low-Quality Training Data
The quality of an AI model’s training data significantly influences its performance. If the model is trained with insufficient, outdated, or low-quality data, it may struggle to generate accurate, relevant responses. It might hallucinate by producing outputs based on patterns it thinks it has identified in its training data, but these patterns may not accurately represent the task at hand or the real-world context.
Overfitting
Overfitting is a common issue in machine learning where a model performs well on its training data but poorly on new, unseen data. Overfit models are typically complex, with many parameters fine-tuned to capture noise or random fluctuations in the training data. This results in memorizing the training data and failing to generalize to new data.
Biases in Training Data
Bias in training data is another significant factor that can lead to AI hallucinations. If the data used to train an AI model is biased, the model will likely reproduce and amplify these biases in its responses. For instance, if a language model is trained on text data that over-represents a particular demographic group, it might generate biased outputs that favor that group.
Model Misinterpretation
Finally, model misinterpretation can also cause AI hallucinations. This can occur when an AI system incorrectly interprets the patterns it identifies in its training data. For example, a model might identify a correlation in its training data and interpret this as a causative relationship, leading to inappropriate or inaccurate outputs.
It is essential to comprehend the reasons behind AI hallucinations as they are pivotal in developing more dependable and trustworthy AI systems. By dealing with issues like data quality, bias, overfitting, and model interpretation, we can reduce the danger of hallucinations and augment the performance of AI systems.
Aivia's Approach to Understanding and Mitigating AI Hallucinations
To prevent hallucinations in AI-generated content, it is crucial to have a human supervise the process during both training and content creation. To learn more about the human-in-the-loop approach to mitigating AI systems’ hallucinations, ensure to watch our video, which you can find here.