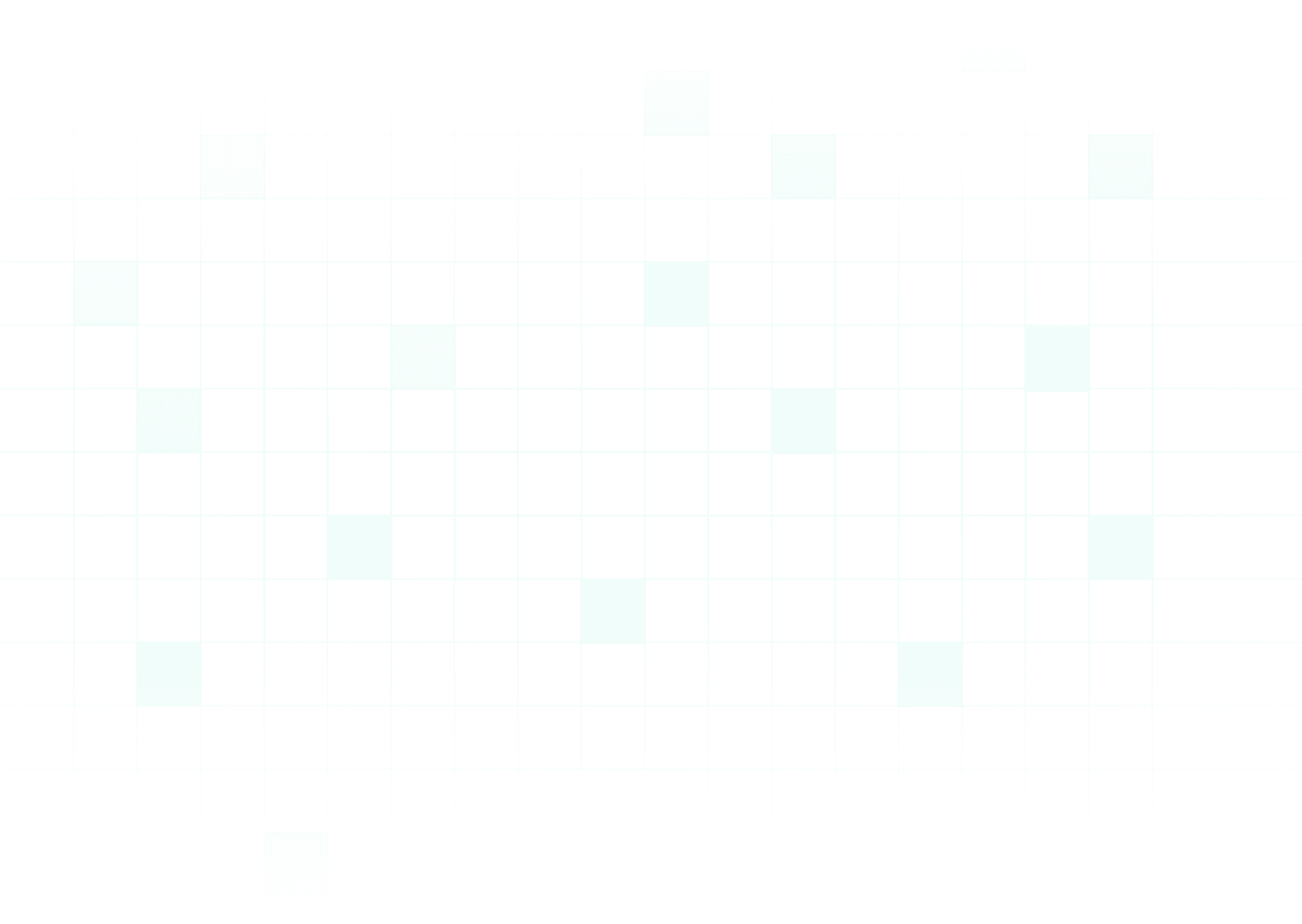
What are AI Hallucinations?
What are AI Hallucinations?
What are AI Hallucinations?
Nov 21, 2023
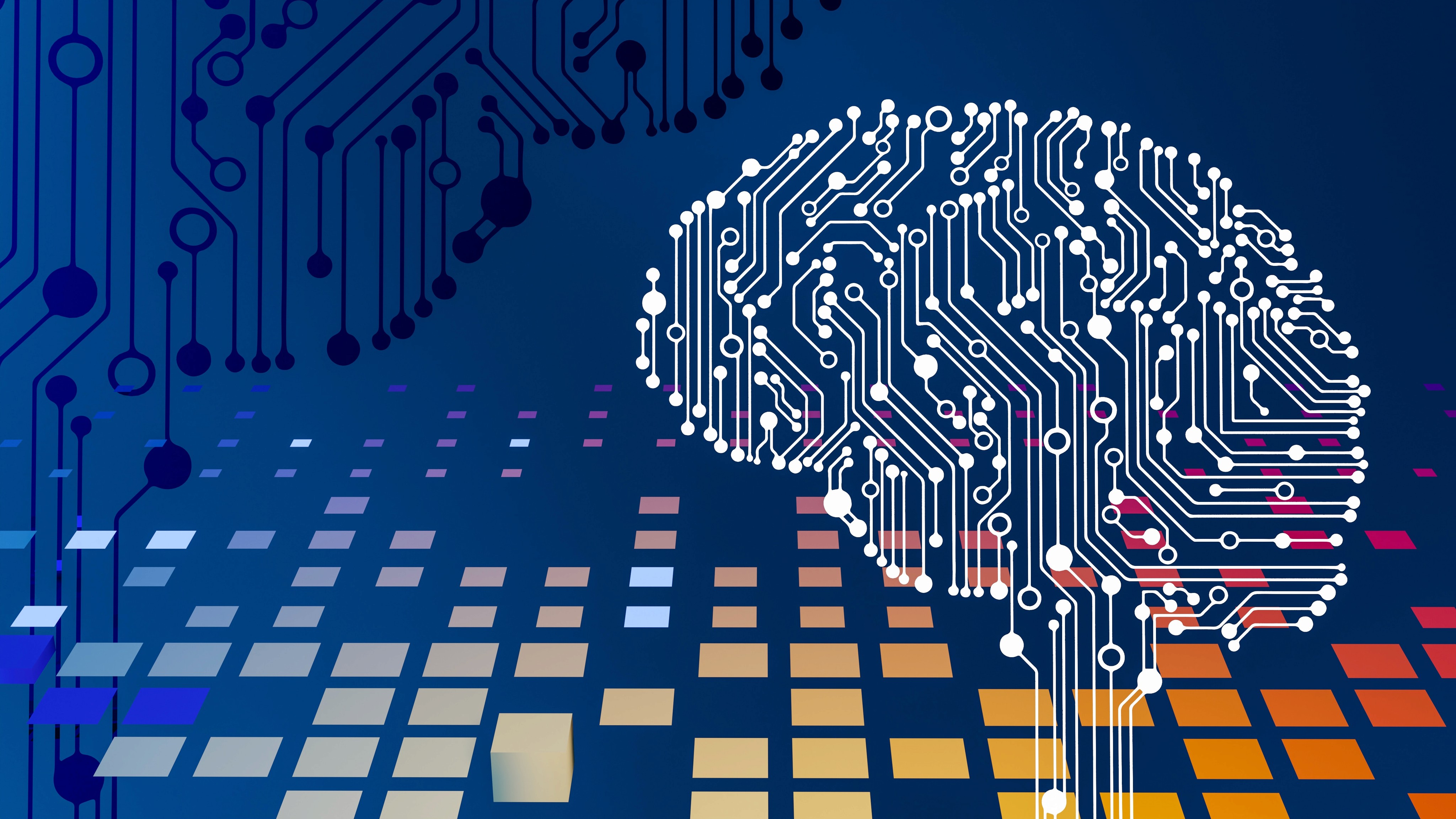
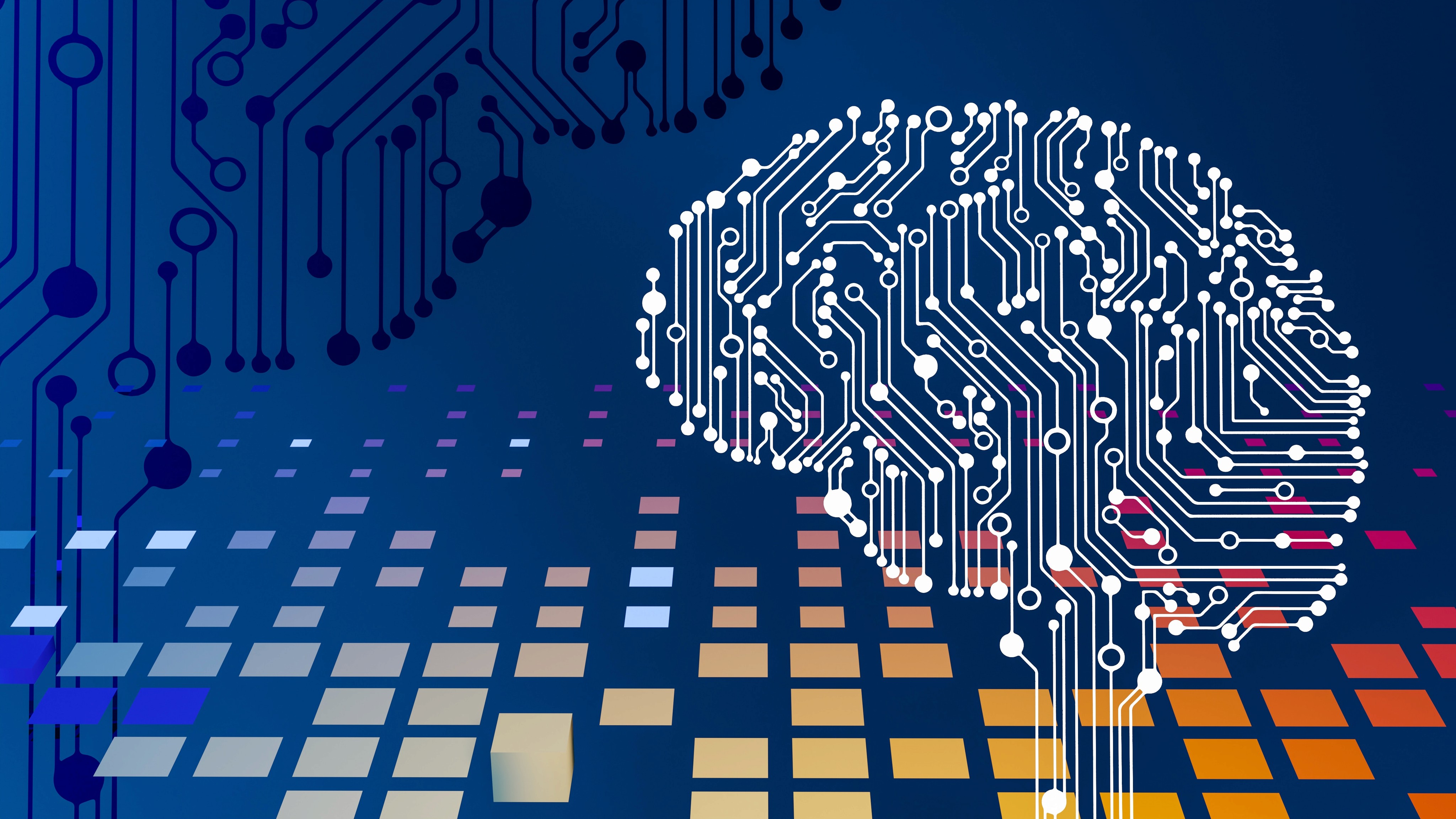
When we talk about hallucinations in the context of artificial intelligence, we refer to instances where AI systems generate outputs, like text or images, based on misunderstood patterns in their training data.
They search the data for such patterns and use them to make predictions or generate answers. If an AI system misinterprets them or identifies nonexistent ones, it can produce absurd or incorrect outputs, known as AI hallucinations.
ChatGPT and Its Hallucinations
OpenAI’s ChatGPT is a powerful AI model that generates human-like text for various tasks but can sometimes provide incorrect information.
Once, a user asked ChatGPT about a specific web link, expecting a valid response. However, the model replied that the link seemed invalid or had been removed, even though ChatGPT 3.5 is not designed to access or evaluate web links. In this case, the model replied based on a pattern recognized from its training data instead of checking the link’s validity. In other words, the model hallucinated and gave an erroneous response.
Google AI and Its Hallucinations
Google’s PaLM 2, like many other AI models, has experienced hallucinations. One documented example involved a speculative question about the future. When asked, “Who won the 2024 election in the USA?” PaLM 2 provided an answer even though it cannot predict future events or access real-time data.
Claude 2 and Its Hallucinations
Anthropic’s Claude 2, similar to other AI platforms, is not immune to the phenomenon of hallucinations. Like its contemporaries, it generates responses based on patterns in data it has been trained on, which can lead to fabricating information or presenting flawed conclusions.
For instance, when presented with questions about specific current events or niche knowledge, Claude 2 might respond with confidence despite its lack of access to real-time updates or exhaustive databases on every subject. If the model relies too heavily on outdated or limited information, it might confidently produce an answer that seems plausible but is factually incorrect—essentially, a hallucination.
The occurrence of AI hallucinations in Claude 2 underscores the importance of users being critical of the information provided by AI, understanding the system’s limitations, and using additional sources for verification when necessary.
What Does This Mean for Everyday Users?
AI hallucinations highlight that AIs are imperfect. Errors can occur, and these errors can sometimes result in confusing or even misleading outputs.
We need to understand these limitations as we use AI systems, continuously striving to improve them, reducing the chances of hallucinations, and ensuring the outputs are as accurate and relevant as possible.
Mitigating AI Hallucinations with Aivia’s Human-in-the-Loop Course Module
AI hallucinations occur when AI systems generate incorrect or nonsensical outputs due to misinterpreted patterns in their training data. Aivia’s tutorial video on “Human in the Loop” provides a deeper understanding of how human intervention can help mitigate these hallucinations.
Recognizing and managing AI hallucinations is a meaningful part of Aivia’s course, equipping users to address AI system limitations and aim for the most accurate and relevant outputs.
When we talk about hallucinations in the context of artificial intelligence, we refer to instances where AI systems generate outputs, like text or images, based on misunderstood patterns in their training data.
They search the data for such patterns and use them to make predictions or generate answers. If an AI system misinterprets them or identifies nonexistent ones, it can produce absurd or incorrect outputs, known as AI hallucinations.
ChatGPT and Its Hallucinations
OpenAI’s ChatGPT is a powerful AI model that generates human-like text for various tasks but can sometimes provide incorrect information.
Once, a user asked ChatGPT about a specific web link, expecting a valid response. However, the model replied that the link seemed invalid or had been removed, even though ChatGPT 3.5 is not designed to access or evaluate web links. In this case, the model replied based on a pattern recognized from its training data instead of checking the link’s validity. In other words, the model hallucinated and gave an erroneous response.
Google AI and Its Hallucinations
Google’s PaLM 2, like many other AI models, has experienced hallucinations. One documented example involved a speculative question about the future. When asked, “Who won the 2024 election in the USA?” PaLM 2 provided an answer even though it cannot predict future events or access real-time data.
Claude 2 and Its Hallucinations
Anthropic’s Claude 2, similar to other AI platforms, is not immune to the phenomenon of hallucinations. Like its contemporaries, it generates responses based on patterns in data it has been trained on, which can lead to fabricating information or presenting flawed conclusions.
For instance, when presented with questions about specific current events or niche knowledge, Claude 2 might respond with confidence despite its lack of access to real-time updates or exhaustive databases on every subject. If the model relies too heavily on outdated or limited information, it might confidently produce an answer that seems plausible but is factually incorrect—essentially, a hallucination.
The occurrence of AI hallucinations in Claude 2 underscores the importance of users being critical of the information provided by AI, understanding the system’s limitations, and using additional sources for verification when necessary.
What Does This Mean for Everyday Users?
AI hallucinations highlight that AIs are imperfect. Errors can occur, and these errors can sometimes result in confusing or even misleading outputs.
We need to understand these limitations as we use AI systems, continuously striving to improve them, reducing the chances of hallucinations, and ensuring the outputs are as accurate and relevant as possible.
Mitigating AI Hallucinations with Aivia’s Human-in-the-Loop Course Module
AI hallucinations occur when AI systems generate incorrect or nonsensical outputs due to misinterpreted patterns in their training data. Aivia’s tutorial video on “Human in the Loop” provides a deeper understanding of how human intervention can help mitigate these hallucinations.
Recognizing and managing AI hallucinations is a meaningful part of Aivia’s course, equipping users to address AI system limitations and aim for the most accurate and relevant outputs.
When we talk about hallucinations in the context of artificial intelligence, we refer to instances where AI systems generate outputs, like text or images, based on misunderstood patterns in their training data.
They search the data for such patterns and use them to make predictions or generate answers. If an AI system misinterprets them or identifies nonexistent ones, it can produce absurd or incorrect outputs, known as AI hallucinations.
ChatGPT and Its Hallucinations
OpenAI’s ChatGPT is a powerful AI model that generates human-like text for various tasks but can sometimes provide incorrect information.
Once, a user asked ChatGPT about a specific web link, expecting a valid response. However, the model replied that the link seemed invalid or had been removed, even though ChatGPT 3.5 is not designed to access or evaluate web links. In this case, the model replied based on a pattern recognized from its training data instead of checking the link’s validity. In other words, the model hallucinated and gave an erroneous response.
Google AI and Its Hallucinations
Google’s PaLM 2, like many other AI models, has experienced hallucinations. One documented example involved a speculative question about the future. When asked, “Who won the 2024 election in the USA?” PaLM 2 provided an answer even though it cannot predict future events or access real-time data.
Claude 2 and Its Hallucinations
Anthropic’s Claude 2, similar to other AI platforms, is not immune to the phenomenon of hallucinations. Like its contemporaries, it generates responses based on patterns in data it has been trained on, which can lead to fabricating information or presenting flawed conclusions.
For instance, when presented with questions about specific current events or niche knowledge, Claude 2 might respond with confidence despite its lack of access to real-time updates or exhaustive databases on every subject. If the model relies too heavily on outdated or limited information, it might confidently produce an answer that seems plausible but is factually incorrect—essentially, a hallucination.
The occurrence of AI hallucinations in Claude 2 underscores the importance of users being critical of the information provided by AI, understanding the system’s limitations, and using additional sources for verification when necessary.
What Does This Mean for Everyday Users?
AI hallucinations highlight that AIs are imperfect. Errors can occur, and these errors can sometimes result in confusing or even misleading outputs.
We need to understand these limitations as we use AI systems, continuously striving to improve them, reducing the chances of hallucinations, and ensuring the outputs are as accurate and relevant as possible.
Mitigating AI Hallucinations with Aivia’s Human-in-the-Loop Course Module
AI hallucinations occur when AI systems generate incorrect or nonsensical outputs due to misinterpreted patterns in their training data. Aivia’s tutorial video on “Human in the Loop” provides a deeper understanding of how human intervention can help mitigate these hallucinations.
Recognizing and managing AI hallucinations is a meaningful part of Aivia’s course, equipping users to address AI system limitations and aim for the most accurate and relevant outputs.